Insurance fraud delays honest claims, raising costs for everyone. Machine learning is speeding up fraud detection, improving claim accuracy and efficiency for better customer service.
Imagine filing a claim after a minor fender bender, expecting a smooth process—only to hear, “We need more time to investigate.”
Frustrating, right?
Fraudulent claims are often to blame for these delays, forcing insurers to scrutinize every detail. It’s not just their headache; fraud drives up costs for everyone, leading to higher premiums and slower payouts for honest customers.
Insurance companies are juggling thousands of claims daily, and with fraud becoming more sophisticated, manual detection is no longer enough. Fortunately, machine learning (ML) is transforming how fraud is identified and stopped, helping insurers stay one step ahead while improving service for honest policyholders.
The Real Cost of Insurance Fraud
Fraud in insurance claims takes many forms, from staged accidents to falsified injury reports. These fraudulent activities drain company resources, and the costs trickle down to consumers. It’s estimated that 10-30% of all claims involve some form of fraud, resulting in billions in losses each year.
Fraud creates operational slowdowns and impacts customer trust. Delayed or disputed claims frustrate customers, causing some to switch providers. As fraud becomes more elaborate, insurance companies need smarter solutions. Traditional rule-based systems—like flagging any claim over a certain amount—aren’t agile enough.
Worse, they can mistakenly target legitimate claims, leading to unnecessary delays. In an increasingly digital world, insurers need technology that can adapt to new fraud patterns quickly. That’s where machine learning comes in.
How Machine Learning Detects Fraud Faster
Think of machine learning as a super-detective for data. It can process massive amounts of information in minutes, scanning for patterns and anomalies that might take a human investigator weeks to notice.
Here’s why ML is a game-changer for insurance fraud detection:
- Speed: Algorithms can review claims instantly, reducing delays.
- Accuracy: ML identifies patterns invisible to the human eye, minimizing errors.
- Scalability: ML systems handle thousands of claims simultaneously, improving efficiency.
Instead of rigid rules, ML models learn from historical data. They spot subtle patterns that evolve, making them more effective in catching sophisticated fraud attempts. This adaptability helps insurers keep payouts fair without slowing down the process for genuine claims.
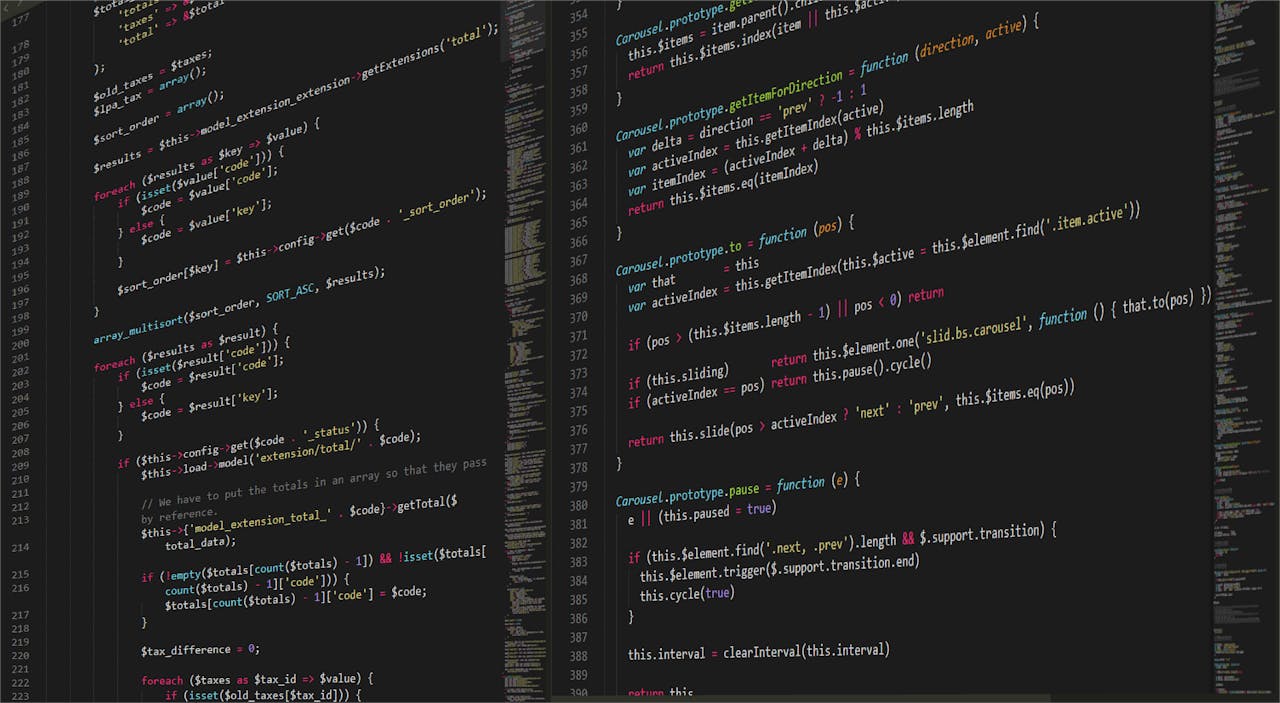
Top Machine Learning Models for Fraud Detection
Different ML models bring different strengths to fraud detection. Here’s how they stack up:
Model | Accuracy | Precision | Recall | F1-score |
XGBoost | 89% | 91% | 89% | 89% |
Support Vector Machine (SVM) | 86.5% | 67% | 75% | 75% |
Logistic Regression (LR) | 72% | 65% | 88% | 74% |
Decision Trees (DT) | 63.6% | 60% | 63% | 60% |
XGBoost stands out with 89% accuracy and 91% precision, making it ideal for insurers who need both speed and reliability. It minimizes false positives (flagging honest claims) while also reducing false negatives (letting fraud slip through).
Meanwhile, SVM performs well with smaller datasets but can be resource-intensive. Logistic Regression offers high recall, making it suitable for sectors like health insurance, where catching every potential fraud case is crucial.
Decision Trees, though easy to interpret, struggle with more complex data, highlighting the importance of choosing the right tool for the job.
Challenges of Using Machine Learning for Fraud Detection
Despite its potential, ML comes with some challenges insurers must navigate:
- Data Imbalance: Fraud makes up a small portion of claims, making it tricky for models to learn effectively. Using techniques like the Synthetic Minority Over-sampling Technique (SMOTE) can help balance the dataset, though it risks generating overfitted models.
- Explainability: Advanced models like XGBoost are often seen as “black boxes.” Insurers need tools that explain ML decisions clearly to earn the trust of stakeholders and regulators.
- Real-Time Detection Limits: Although ML models perform well with historical data, real-time fraud detection remains a hurdle. Insurers need fast, reliable infrastructure to process claims instantly and prevent fraud before payouts occur.
These challenges highlight the need for continuous improvement in ML systems. By investing in better models and infrastructure, insurers can unlock the full potential of fraud detection technologies.
The Future of Fraud Detection with Real-Time Solutions
The future of fraud detection lies in real-time analysis. Imagine submitting a claim and receiving a decision within seconds—powered by ML models working behind the scenes. Streaming data solutions are making this a reality, helping insurers spot and block fraud in real-time.
Ensemble models, which combine multiple algorithms, offer even greater accuracy. For example, pairing XGBoost with feature engineering techniques can improve performance while enhancing model transparency.
Additionally, the rise of explainable AI tools will allow insurers to clarify how decisions are made, building trust with policyholders and regulators alike.
These advancements aren’t just about catching fraud—they also streamline operations, reduce costs, and improve the customer experience.
How Machine Learning Helps Insurers Save Time and Money
Machine learning is changing the way insurers detect and prevent fraud, offering tools that are faster, smarter, and more efficient than traditional methods. However, success depends on choosing the right model and continuously refining detection systems.
Key Takeaways
- XGBoost is the top performer, balancing accuracy, precision, and recall.
- SVM excels in smaller datasets but requires more computational resources.
- Real-time detection systems are the future—insurers must invest in streaming data tools.
- Transparency and explainability are crucial for building trust with customers and stakeholders.
Practical Advice for Insurers
- Explore ensemble models to maximize accuracy and reliability.
- Invest in explainable AI tools to ensure transparency in decisions.
- Adopt multiple ML models to cover different types of fraud scenarios.
- Develop real-time fraud detection capabilities to stay ahead of evolving fraud tactics.
The message is clear: fraudsters aren’t slowing down, and neither can insurers. With the right tools and strategies, insurers can stay ahead of fraud, protect their bottom line, and deliver better service to their customers.
What Should Insurers Do Next?
If you’re in the insurance industry, the time to embrace ML-powered fraud detection is now. The sooner you integrate these tools, the faster you’ll reduce risks, cut costs, and gain a competitive edge.
Fraud detection is no longer just about catching bad actors—it’s about improving customer trust and operational efficiency. By investing in machine learning, insurers can ensure they stay resilient and customer-focused in a fast-changing world.
RELATED TOPICS
- How To Craft The Perfect Data Loss Prevention Strategy
- Why Incident Response Planning is Critical for Cybersecurity
- What is an Infosec Audit, Why Does Your Company Need One?
- Unravelling Retirement Banking Scams – How To Protect Yourself
- Protect your identity from crooks with AI-powered Identity Guard