Owing to its relative nascency, the realm of artificial intelligence (AI) has continued to undergo a rapid transformation while being faced with several developmental challenges. Most recently, the issue of a one-size-fits-all approach of traditional large language models (LLMs) has become increasingly prominent — especially as different industries have sought out more precise, efficient, and contextually aware AI solutions.
Current generalist models such as Perplexity, ChatGPT, Claude, etc while undoubtedly impressive in their overall scope of operation and knowledge, often fall short when it comes to solving nuanced and complex challenges specific to domains like robotics, biotechnology, and materials science.
In fact, there is enough statistical data highlighting this need for such specialized AI systems. For instance, the robotics market is projected to reach a massive valuation of $97 billion by 2029, with its associated software segment alone expected to expand to $80 billion by 2032.
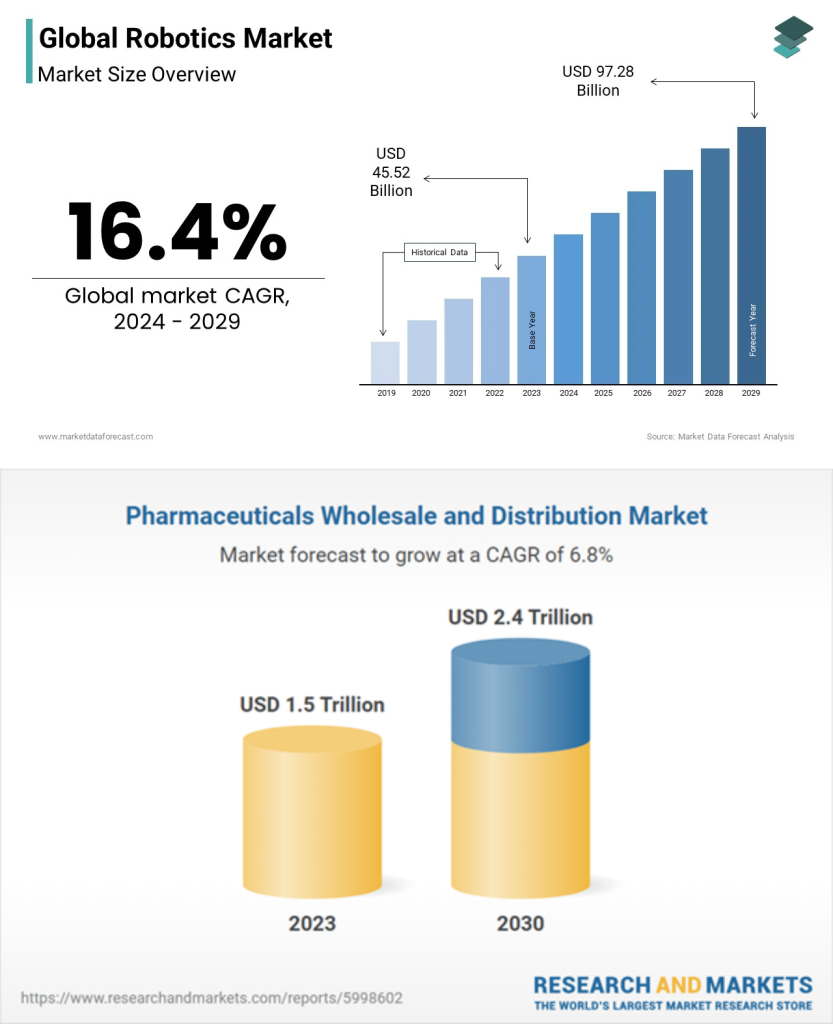
Similarly, the materials science and drug discovery sectors have also witnessed exponential growth, with markets for advanced materials and pharmaceuticals projected to reach $121 billion and $2.4 trillion respectively in the mid-to-long term.
ASI Train and its novel approach to domain-specific AI
On November 26, 2024, the Artificial Superintelligence (ASI) Alliance — a conglomerate consisting of industry giants like SingularityNET, Fetch.ai, and Ocean Protocol — introduced ‘ASI Train,’ a platform offering a strategic shift from generalist AI models to highly specialized, domain-specific systems.
Its inaugural model, Cortex, is designed to be a $100 million brain-inspired robotics framework demonstrating how targeted AI can revolutionize industries by addressing their unique computational and analytical requirements.
At its core, ASI Train leverages a decentralized framework that democratizes AI development and ownership. By utilizing Fetch.ai’s Autonomous Inference Model (AIM) Agents, the platform creates a collaborative ecosystem where researchers, investors, and technology enthusiasts can actively work with each other.
This has been done in order to not only distribute the system’s computational and financial burden but also ensure that the benefits of advanced AI are shared equitably among all of the involved stakeholders.
The proof is in the pudding
From the outside looking in, ‘Cortex’ serves as a proof of concept for ASI Train’s operational methodology. Inspired by the day-to-day functioning of the human brain, the model seeks to create robots that are not merely reactive but contextually aware and adaptive.
By combining vision-language-action data sets with brain-inspired architectures, Cortex can help propel robotics to another level; especially since it does not rely on preprogrammed instructions for its operational autonomy.
The potential impact of such specialized tools extends across multiple critical sectors. In materials science, for instance, the platform can accelerate high-throughput screening processes, thus dramatically reducing the computational limitations that have currently bottlenecked discoveries in energy storage, electronics, and nanotechnology.
Similarly, within the drug discovery landscape, ASI Train’s approach promises to transform molecular design by improving the efficiency of identifying viable drug candidates. By implementing algorithms that can accurately predict protein-ligand interactions, the platform could significantly reduce the time and astronomical costs associated with pharmaceutical research.
To this point, it bears mentioning that developing a single new drug can currently range anywhere up to a whopping $2.3 billion, making any efficiency gain potentially transformative for the industry.
The future of AI is unfolding as we speak
In addition to its aforementioned capabilities, ASI Train comes replete with a unique tokenomics structure wherein users can stake $FET to gain ownership of specific AI models, creating a unique value proposition where community members are not just passive consumers.
In fact, such a decentralized/non-local setup allows for secondary market trading of AI model assets, effectively turning cutting-edge technological development into a collaborative, economically engaging endeavor. On the subject, Humayun Sheikh, CEO of Fetch.ai and chairman of the ASI Alliance was recently quoted as saying:
“By combining domain-specific models like ‘Cortex’ with decentralized ownership, we’re creating a DeSci ecosystem where individuals support groundbreaking technology and share value creation.”
Lastly, it is estimated that Cortex’s initial 12-14 week training period will begin by the end of December and will most likely generate an annual revenue exceeding $10 million (from customers ranging from educational institutions to warehouse companies to robotics).
Therefore, looking ahead, the ASI Alliance has set its sights on expanding its portfolio with additional AI models spanning domains like biotechnology, quantum science, and even space tech.